Quality for Data Systems - Let's Talk Strategy
Data is a crucial asset to every business and organization and has sparked fields such as data analytics, data science, and machine learning or artificial intelligence. Organizations have invested heavily in engineering teams to help them store, organize, transform, and understand their data.
Data Engineers build various systems to collect and store data. While there are numerous project configurations, these are the most common:
- Extract-Transform-Load (ETL) or Extract-Load-Transform (ELT)
- Migration
- Streaming
- Artificial Intelligence/Machine Learning
Incorrect data affects a business's ability to serve its customers, resulting in a loss of credibility and revenue, customer dissatisfaction, and potential compliance issues. A holistic test strategy can help teams build quality into their systems and working methods.
This post aims to reshape our thinking about quality engineering for data projects. We'll provide a holistic test strategy and introduce testing methods to ensure quality in many ways. We will not dive deep into each topic or section but instead offer a guideline where you can approach creating a quality strategy tailored to your project and systems.
Quality Strategy Overview
The data pipelines built by data engineers are just software. Software that can be tested and deployed just like any other. Data is moved and manipulated with these systems. But applying a systematic quality assurance strategy or process is relatively new within the industry.
The concepts are simple:
⚡️ The end product consists of the data pipelines (functionality) and the data itself. So we must test both.
⚡️ Ensure quality during development and in production.
A critical fact – every recommendation for software engineering quality best practices applies to data engineering! That's writing good quality code with testability in mind, branching strategies, code reviews, unit testing (read on) and more.
Let's present essential quality validations to consider:
Testing Type | Description | Notes |
---|---|---|
Unit | Code is written to test a specific unit of code | Don't confuse this with a manual functional test you perform on one item. We're talking traditional unit tests, where you write code to test another piece of code using a unit testing library. |
Functional | Testing any functionality, logic, or behavior | There are various methods to test functionality, many of which can be automated. |
Data | Validating that the data meets expectations | Expectations can come from various people, but mainly from the business. |
Security | Ensuring the proper access controls and security measures are in place for both pipelines and data | |
Performance | Validating pipelines are performing as expected in terms of speed and/or load | |
Data standards adherence | Adhering to agreed-upon naming, data type, and schema standards set by the team | Ensuring suitable data types are used under the right circumstances. |
Code quality & consistency | Implementing and adhering to consistent code style and standards | Applicable to any code, even SQL, for example. |
We're going to focus on Functional and Data testing in this post.
Quality During Development
During development, we're working in non-production environments (hopefully!) As is standard, functional testing is appropriate at this stage.
However, data testing may not be applicable, as we may be working with test data from test environments. An issue in data quality is difficult to differentiate between something real vs a result of incorrect data specific to the test environment. With that said, there could be data tests we can perform if they pertain to any functionality or code we write. So, assess this carefully for your system.
While we may not test the data during development, we can test our tools and frameworks that will run in production to validate data quality. In fact, lower environments are best for this case since we have more control of the data and can add what we need.
Quality In Production
In production, our systems are live and functioning. We're also dealing with actual data that is needed by end-users. So we must focus on both functional and data testing.
Functional testing ensures everything is working as expected, and data testing ensures data issues don't break our pipelines or cause erroneous results for end users. Often, the same tools and methodologies we use for testing during development apply to testing in production.
Another essential quality dimension to consider is observability. How do we know if our pipelines and data in production have an issue? Think about monitoring your system in production. We collect metrics, build dashboards where needed, monitor the metrics, and alert when a threshold is exceeded. The team then acts and remediates the issue. Perhaps reloading or fixing specific data sets or re-running pipelines.
Confusing Functional & Data Testing
If you search "data testing," the majority of results will be about "data quality testing" referencing these dimensions:
- Completeness
- Integrity
- Uniqueness
- Accuracy
- Consistency
- Timeliness
These are all valid. The challenge is that they are data characteristics that, from a typical quality engineering perspective, touch upon functional and data tests. For example, completeness and timelines can be considered part of functional testing. This can be confusing because we focus on the terms rather than creating a proper strategy tailored to our project.
Functional Tests
Pipelines and code can constantly change. We get new requirements, or we may need to fix bugs. Before releasing new or updated code to production, we need to ensure quality and that we didn't unintentionally break anything.
Are pipelines working as we expect? Are they successfully moving data from one location to another? Beyond the pipelines, there could be transformation logic as well. Is this logic working as expected?
Keep in mind the practice of shifting quality left where we implement quality practices as early as possible within the Software Development Life Cycle. In terms of automated testing, follow the Test Pyramid. So if you use a programming language that provides a unit testing library, most of your functional testing can be covered with unit tests.
As you move up the Test Pyramid and add component, integration, and end-to-end tests, you may need setup test data to run your automated tests. One gotcha teams face is using massive data sets for testing. You don't need 1 million rows to validate a requirement. See if you can create a baseline test data set for each level of testing. This can reduce your test data size, decrease the test execution time, and enable automated testing with a repeatable set.
Data Tests
The data we move has an end-user in mind. It serves a purpose for someone. And that someone usually has requirements for their data. We must ensure it's high quality and meets the user's expectations.
From an engineering perspective, data can come from multiple sources in multiple forms. We must understand it as it's being ingested and flowing through our system. That helps us set data quality expectations throughout our pipelines. For example, we may not want a column to contain nulls because we know that will break our logic or cause reporting issues downstream.
Profiling the source data to understand the possible values, types, distributions, and relationships is crucial. This helps us realize possible values and with setting appropriate data expectations. Or it may raise some critical questions for the business or data providers to answer. We should also understand how the data will be used to further our knowledge.
Test Methodologies
There are various ways to perform functional and data testing. And these methods are intertwined. The majority can cover both, but some are better than others. Confusing, I know.
The key is to understand the pros and cons of each approach and pick the ones that work for you. Here's a quick table of each method and what it covers. We'll dive into each one in the subsequent sections.
Method | Coverage | Best For |
---|---|---|
Unit tests | Functional | Functional |
Side-by-side comparisons | Functional & Data | Functional |
Column-level validations | Functional & Data | Data |
Data profiling/metadata comparisons | Functional & Data | Functional |
Unit Tests
In any test strategy, unit tests are crucial. They're fast and reliable, and we can control every parameter.
Let's get on the same page about defining a unit test. It is not when we manually test a story or feature. It's when we write code to test other code (usually via a unit testing library like pytest) without spinning up our solution. It's testing a specific function, for example. Validating code at the lowest level.
Of course, these should run in your build pipelines and gate any merge to your main or master branch. Pick the most popular library or module available for your programming language.
Side-By-Side Comparisons
In side-by-side comparisons, we validate that one data set exactly equals another. This can check the schema, counts, and values are equal between expected and actual data sets. However, it's best suited for comparing the values of two data sets (expected vs actual).
As you can imagine, this method can be time and resource intensive if you compare massive amounts of data. So don't use it for testing in production. If you want to validate that all data during a load exactly matched the source, use data profiling or metadata comparisons instead. Or use a hashing comparison approach (although debugging will be difficult).
Naturally, this method is excellent for testing functionality during development. We know exactly what the output should be, so we can set up our test data and expected results as we need.
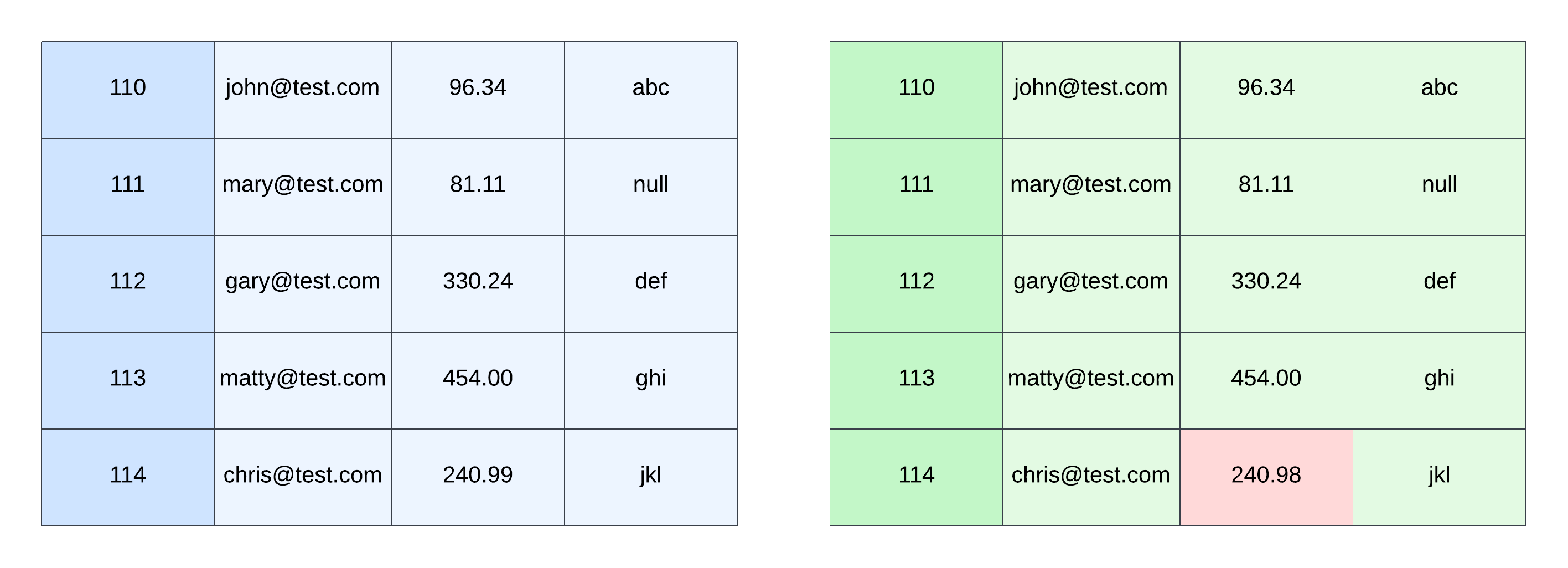
In the above picture, the blue table is our expected result, and the green table is our actual result after pushing our test data through a pipeline. The red cell highlights the difference (or bug) between the two tables.
Few automated testing frameworks or libraries provide the ability for side-by-side comparisons, so many teams build frameworks in-house. This is achievable with any language using readily-available libraries and offers excellent flexibility.
Column-Level Validations
In column-level validations, we check whether a specific column meets specific expectations. But not necessarily against another data set. Instead, it's an inbound test with the expected result defined in the code. For example, are there any rows where the email column is null?
This methodology can be used for functional and data testing, with more skew for data quality testing since it allows for uncertainty in the data. Also, we are usually not checking for a specific value within a particular column and row. We're not comparing against an entire data set, either.
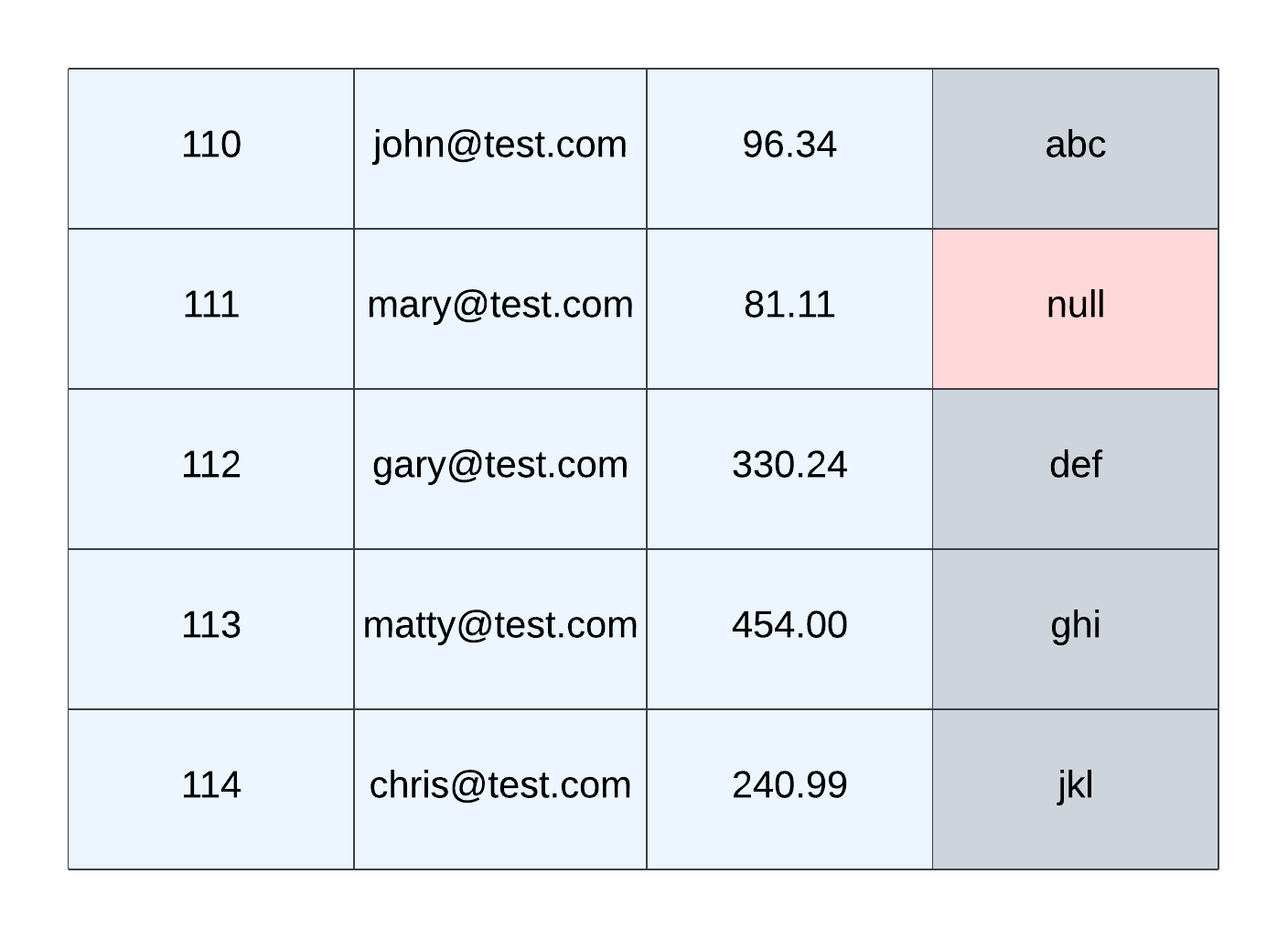
In this diagram, a sample column-level validation checks the last column and ensures no nulls exist.
These tests are usually fast and less resource-intensive than side-by-side comparisons. However, they don't provide as much flexibility for validating a specific scenario or logic. You can be clever about it by controlling your test data, but that may prove difficult. Having said that, you might be able to optimize and use the same tool for functional and data testing during development and production validations.
Many tools and frameworks exist to help facilitate this testing methodology, with Great Expectations and Deequ being the front runners. Some platforms have built this in even, like Databricks with their Delta Live Tables Expectations.
Data Profiling/Metadata Comparisons
Data profiling or metadata comparisons is when we use the characteristics of the data to ensure quality. Some examples include:
- Row and column counts
- Min/max values
- Data types
- Distinct values
This methodology is fast but the least comprehensive, as it doesn't guarantee all the data is accurate. It could be a "good-enough" approximation or a first test before running more extensive validations. It can also be used for observability.